‘Oh my goodness! AI is accelerating in capability even faster now, than it was 6 months ago or 1 year ago. Wow! This is astonishing what the claims of capabilities are! No wonder you are posting so often Bindu! My goodness.’
‘Time to send tweets to Mark Zuckerberg! They question him, and fight him, and he keeps finding a way to fight back.’
“If Llama 3 400B+ can achieve a 96% score on ARC, it would be a remarkable achievement, highlighting its ability to go beyond language and delve into the realm of scientific reasoning.”
Here’s a breakdown of the types of elementary science concepts and reasoning that an AI model scoring highly on the ARC Challenge might be able to handle:
Basic Physics:
Basic Chemistry:
Basic Biology:
Reasoning Abilities Demonstrated:
The skills needed to excel on the ARC Challenge, especially with a high score like 96, suggest that the AI model is likely developing capabilities relevant to material science and math as well.
Here’s how those areas connect:
Material Science:
Math:
Important Caveat:
The ARC-Challenge:
-
Understanding ARC-Challenge: The AI2 Reasoning Challenge (ARC) is a dataset containing grade-school level multiple-choice science questions. These questions are designed to be challenging and require advanced reasoning skills beyond simple pattern matching. The “Challenge“ set in ARC consists of particularly difficult questions that often require complex reasoning, external knowledge, or both.
-
25-Shot Learning Context: The score of 96 in a “25-shot” setting indicates that the model was provided with 25 examples of similar questions along with their answers before it was tested. This allows the model to “learn” or “adapt” to the style and complexity of the ARC questions in a limited context, enhancing its ability to predict answers correctly when faced with new, unseen questions.
-
High Score Interpretation: Achieving a score of 96 in this context suggests that the model correctly answered 96% of the ARC-Challenge questions. This is an exceptionally high score, indicating a very strong performance in understanding and applying complex reasoning to answer science questions correctly.
-
Comparative Performance: This score allows us to compare the efficacy of different models or different training methods. For instance, comparing this to other tasks or models might provide insights into how well this particular model (Meta Llama3 – 400b+) performs in contexts requiring complex reasoning and knowledge integration, as opposed to other tasks like MMLU or Big-bench, which might test different capabilities.
-
Implications for AI and Education: Such high performance on a complex task like the ARC-Challenge suggests potential applications in educational technologies, where AI can assist in teaching complex subjects or even help in designing educational content and testing.
-
Benchmarking and Model Development: For developers and researchers, this score is crucial for benchmarking the capabilities of large language models. High scores in ARC-Challenge can drive further improvements in AI models, particularly in enhancing reasoning and comprehension abilities.
Additional Notes:
Note #1: The reported capabilities of LLaMA 3 are impressive, but it’s essential to wait for official information and independent verification.
Note #2: Even at this early stage, AI’s growing capacity for scientific reasoning is a promising sign for its potential to contribute to various fields.
Note #3: Keep these links in mind, and don’t forget to check periodically this documentation and the download links for this model!!! (Keep a backup safe! And learn how to use it to engineer products and the future!)
Note #4: How do we run this thing offline!?! Holy! Where’s NVIDIA when you need a good computer?!?
Research and Download Links
Title: “Introducing Meta Llama 3: The most capable openly available LLM to date” Meta AI Blog” https://ai.meta.com/blog/meta-llama-3/
Title: “Build the future of AI with Meta Llama 3″ Llama 3 Information” https://llama.meta.com/llama3/
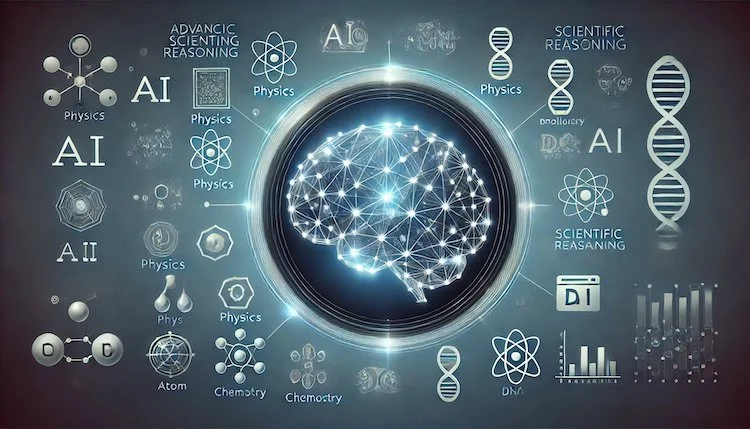
To see our Donate Page, click https://skillsgaptrainer.com/donate
To see our YouTube Channel, click https://www.youtube.com/@skillsgaptrainer